Image public
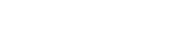
An essential step of risk assessment methodologies in subsurface activities is to simulate multiple possible realizations for the spatial distribution of the lithological facies.
Digital transformation
towards geosciences 4.0