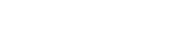
Knowledge is massively present in a very large and always-growing number of documents such as scientific literature, patents, internal reports, etc. Efficiently searching for relevant information within this mass of unstructured data is often a time-consuming prerequisite of scientific tasks.
The main motivation of this work was to create an AI-based system, able to identify among a large corpus of documents which ones are relevant to answer specific scientific questions and to automatically extract pieces of information related to these questions.
A knowledge base containing the information from the corpus was built and is questionable. To do so, several steps were necessary. Firstly, an ontology embedding entities and relations to fit our domain of interest was built. Then predictive AI models were trained, using a limited number of annotated documents, to recognize the ontology’s elements. Applying those models to the full corpus has enabled to extract and store its substantial core within a knowledge base. One last step has consisted in building a natural language, AI-based, querying tool to interrogate the knowledge base.
This methodology was successfully applied on a set of documents in the field of petroleum exploration, in order to answer various questions on source rocks around the globe (e.g. ages, lithology, maturity…) A prototype web-application enables to naturally query the knowledge base as well as navigate within the results.
This methodology could be applied on another corpus of documents or focusing on different subjects. The knowledge base could also be extended using information coming from additional structured data to give more complete answers.