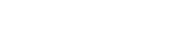
Identification of lithological types from rock samples is cornerstone in many subsurface activities, for instance with field samples or well cuttings. Automation would facilitate the processing of large amounts of samples, or provide insights to professionals involved in subsurface operations without a geology background.
In this study, we assessed the potential of Deep Learning technologies to automate the classification of rock samples pictures among lithological classes. A demonstration project was designed using field samples photographs and 12 lithofacies families.
We benchmarked and fine-tuned several reference architectures of convolutional neural networks. We used an initital training set of 2700 images from public websites and private collections, carefully collected and labeled by our geology experts, and several data augmentation techniques.
Tested on new pictures, our best classifier returned the exact facies for 92% of the samples.
This promising result encourages us to run similar projects with more lithofacies families. It also paved the way for other projects at the crossroads of computer vision and geology, notably for core interpretation and thin section analysis.