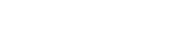
In various contexts, it is important to update existing Machine Learning models with new data in a way that minimizes human effort. For instance, a geologist may want to specialize a data interpretation model for the specific geological features of a given well, field or basin. Similarly, an engineer may want to frequently integrate data coming from field sensors in a model for operations monitoring. However, this task can be quite tedious, notably if the new training data needs to be manually labelled or quality-controlled by experts. Besides, the amount of work required is the same even if most of the new data brings only little added value to the initial model.
Thus, in this project, we aim at leveraging Active Learning methodologies to automatically filter a dataset depending on the value added to an initial model, in order to optimize efficiency in updating this model.
Thanks to Active Learning algorithms, the geologist and the engineer in the examples above will be able to spend time only on the small portion of data actually bringing new information to the existing model.
As a first proof of concept, we implemented an Active Learning strategy for a well-known image classification problem, classically used in Machine Learning benchmarks. In our example, the Active Learning approach results in a 60% decrease in the amount of data needed to obtain the target improvement in accuracy for the base model. Meanwhile, the accuracy gain provided by data automatically selected by Active Learning is 10 points higher than the accuracy gain provided by the same amount of data selected randomly in the whole set.
Our future work on the topic will be to implement Active Learning on geoscience case studies, with the Automated classification of rock samples as the first objective.